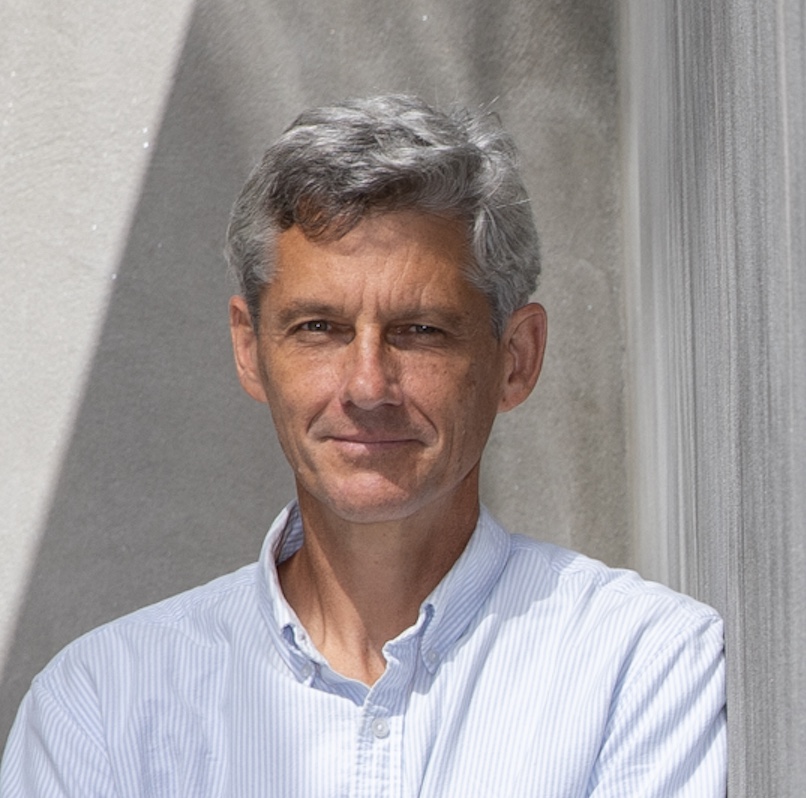 |
Erik G. Learned-Miller
Professor and Chair of the Faculty
The Manning College of Information and Computer Sciences
University of Massachusetts, Amherst
140 Governors Drive, Office 200
Amherst, MA 01003
E-mail: elm at cs.umass.edu
Computer Vision Lab
|
News:
- We have started work on a New Building! Check out the College web pages for architectural drawings and renderings.
- On September 1, 2022, I started my new position as Chair of the Faculty in the Manning College of Information and Computer Sciences. I continue to co-direct the Computer Vision Lab with Subhransu Maji.
|