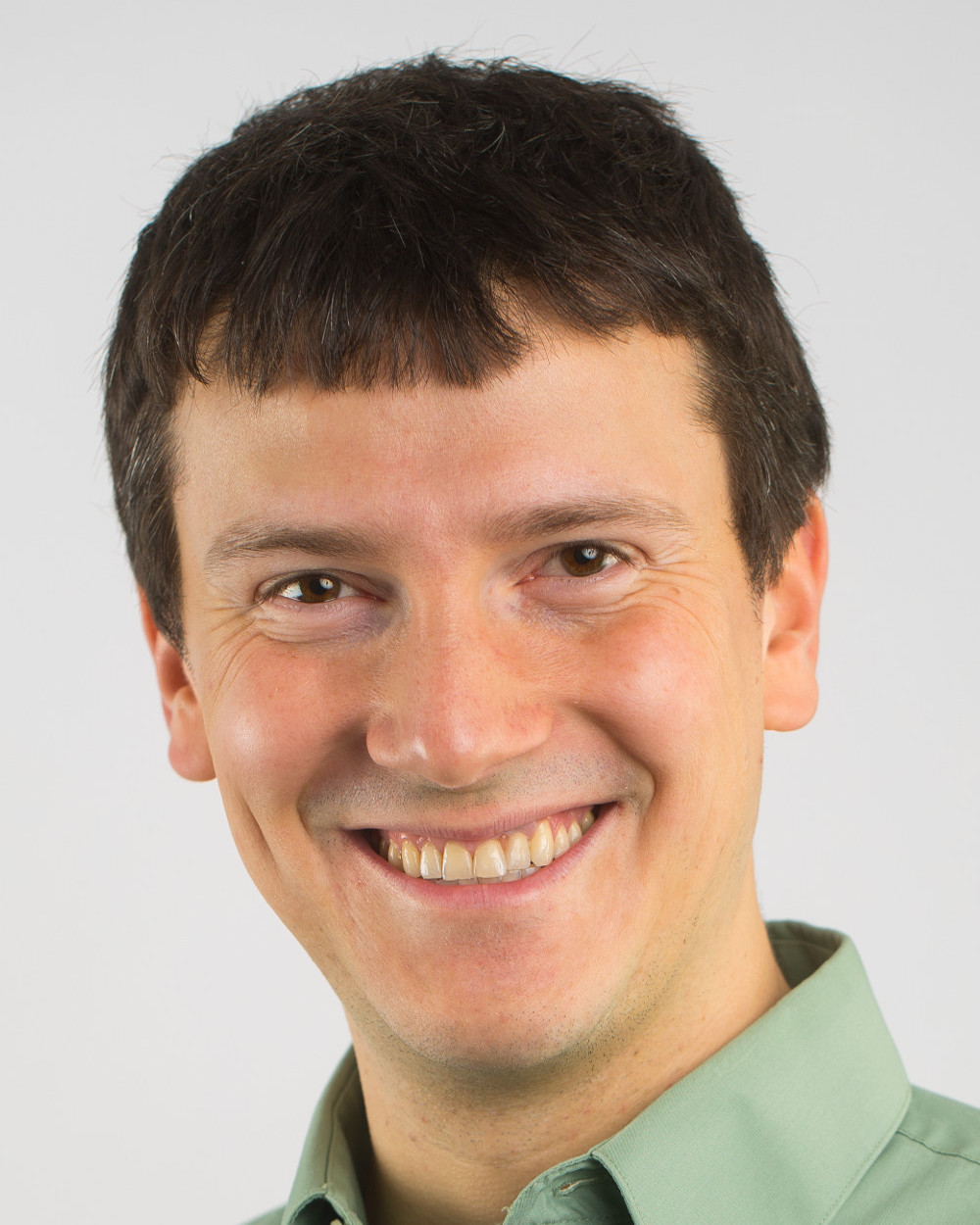
Yuriy Brun
Professor
Manning College of Information & Computer Sciences
140 Governors Drive
University of Massachusetts
Amherst, MA 01003-9264 USA
Email: | |
Office: | 302 |
Phone: | +1-413-577-0233 |
Fax: | +1-413-545-1249 |
- Our ESEC/FSE'15 paper on overfitting in automated program repair won an Test of Time Honorable Mention Award at FSE 2025!
- Our ICSE'25 paper on using LLMs for formal verification won an ACM SIGSOFT Distinguished Paper Award!
- Yuriy elevated to IEEE Fellow!
- Our work on using LLMs to synthesize formal verification proofs published at ICSE 2025.
- Our work on using reinforcement rearning to automate formal verification published at ICSE 2025.
- Our work on attack-resistent image watermarking published at NeurIPS 2024.
- Our work on efficient fine-tuning of LLMs on egde networks published at NeurIPS 2024.
- Our work on whether automated program repair helps developers debug published at ICSE 2024.
- Our work on using large language models to synthesize proofs of mathematical theorems published at ESEC/FSE 2023 and wins an ACM SIGSOFT Distinguished Paper Award! IEEE Spectrum article.
- Our work on understanding how bias affects people's trust published at VIS 2023.
- Manish started a job as an Assistant Professor at Oregon State University!
- Emily started a job as a postdoc at University of California San Diego!
- Manish's and Yuriy's work on using tests and bug reports, together, to improve automated program repair published at ICSE 2023. LASER had a total of 6 papers presented at ICSE 2023, including work on making it easier for software engineers to build systems with fair machine learning and automating formal verification.
- Our work on understing developers published in TOSEM, presented at ICSE 2023.
- Yuriy received a $616K NSF grant on automating formal verification!
- Our work on using identifiers to improve proof synthesis for formal verification published at TOPLAS. Presented at PLDI 2023.
- Yuriy receives a $40K Dolby gift for studying effects of bias in machine learning.
- Yuriy gave a keynote address at MaLTeSQuE 2022.
- Emily's and Yuriy's work on diversity-driven automated formal verification published at ICSE 2022 and wins an ACM SIGSOFT Distinguished Paper Award!
- Google gifts $50,000 for work on mitigating bias in models of ad preferences.
- Yuriy and Phil receive a Google Inclusion Research Award.
- Yuriy and Phil receive a Facebook Building Tools to Enhance Transparency in Fairness and Privacy gift.
- Yuriy receives an Amazon Research Award with $40K funding and $20K AWS credit to support formal verification.
- Yuriy promoted to Professor.
- Alex Sanchez-Stern joins UMass as a postdoctoral researcher!
- Yuriy received the 2021 IEEE Computer Society TCSE New Directions Award.
- Yixue Zhao joins UMass as a CI Fellow postdoctoral researcher!
- Fully-automated formal verification paper accepted to OOPSLA 2020: video.
- Yuriy delivers the ICSSP/ICSSE 2020 Keynote address: video.
- Distributed, privacy-enforcing paper wins SEAMS 2020 Most Influential Paper award! video
- Causal testing paper accepted to ICSE 2020.
- Yuriy promoted to ACM Distinguished Member.
- Safety and fairness guarantees in machine learning paper published in Science.
- Quality of automated program repair paper accepted to IEEE TSE.
- Visualization for system understanding paper accepted to ACM TOSEM.
- Serverless computing paper published at OOPSLA 2019 and is awarded an ACM SIGPLAN Distinguished Paper Award.
- Google gifts $50,000 for work on detecting bias in ads.
- Fair bandits paper published at NeurIPS 2019. Read Fortune summary.
- Automated program repair paper accepted to IEEE TSE.
- Oracle labs gifts $100,000 for work on fairness in rankings.
- Test generation from natural language specifications paper published at ICSE 2019.
- Software fairness paper and demo published at ESEC/FSE 2018.
- Security usability paper published at SOUPS 2018.
- Double blind viewpoint published in Communications of the ACM. News coverage.
- Repair of hard and important bugs paper published in Empirical Software Engineering and presented at ICSE 2018.
- Recovering design decisions paper published in ICSA 2018.
- Our fairness testing paper was the 5th most downloaded SE article in October'17.
- Fairness testing in the news:
EnterpriseTech, Bloomberg, GCN, Co.Design, ACM News, MIT TR Download, UMass. - Automated repair paper published in ASE 2017.
- Fairness Testing paper published in ESEC/FSE 2017 and is awarded an ACM SIGSOFT Distinguished Paper Award.
- NSF funds grant on software fairness testing.
- Yuriy promoted to Associate Professor with tenure.
- Yuriy promoted to Senior Member of both the ACM and the IEEE.
- Yuriy receives the UMass College of Information and Computer Science Outstanding Teacher Award.
- Yuriy receives a Lilly Fellowship for Teaching Excellence.
- Collaborative Design paper published in ICSA 2017 and is awarded the Best Paper Award!.
- Constraint-Aware Resource Scheduling paper published in IEEE Transactions on Systems, Man, and Cybernetics: Systems.
- Behavioral Execution Comparison paper published in ICST 2017.
- Dr. Seung Yeob Shin successfully defended his dissertation. He started at the University of Luxembourg in Fall'16.
- NSF funds medium grant on improving quality of automated program repair.
- Distributed system debugging paper published in Communications of the ACM.
- Performance-aware modeling tool paper presented at ICSE 2016.
- Eureka! Winter'16 program with local Girls Inc. chapter a success!
- Yuriy receives a 2015 Google Faculty Research Award.
- An independent study finds conflict detection work the most industrially relevant of all research published in the top SE conferences in the last five years.
- Yuriy recognized as a UMass Distinguished Teaching Award finalist.
- Two papers on automated API violation discovery presented at ISSRE 2015.
- NSF funds medium grant on improving developer interfaces.
- “Using Simulation...” published in JSS.
- “Repairing Programs...” presented at ASE 2015.
- “Simplifying Development History...” presented at ASE 2015 short paper track.
- “Is the Cure Worse...” presented at ESEC/FSE 2015.
- “The ManyBugs and IntroClass Benchmarks...” featured as the Spotlight Paper in December 2015 by IEEE TSE.
- “Preventing Data Errors...” presented at ISSTA 2015.
- Yuriy receives an ICSE 2015 Distinguished Reviewer Award.
- “Self-Adapting Reliability...” published in IEEE TSE.
- “Reducing feedback delay...” published in IEEE TSE.
- Yuriy receives the NSF CAREER award!
- “Resource Specification...” presented at FASE 2015.
- “Using declarative specification...” published in IEEE TSE.
- “Behavioral Resource-Aware...” presented at ASE 2014.
- “Automatic Mining...” presented at FSE 2014.
- “The Plastic Surgery Hypothesis” presented at FSE 2014.
- Yuriy receives the 2014 Microsoft Research Software Engineering Innovation Foundation Award.
- “Inferring Models of Concurrent...” presented at ICSE 2014.
- “Mining Precise Performance-Aware...” presented at ICSE NIER 2014.
- Yuriy receives the 2013 IEEE TCSC Young Achiever in Scalable Computing Award.
- “Early Detection...” featured as the Spotlight Paper in October 2013 by IEEE TSE.
- “Making Offline Analyses Continuous” presented at ESEC/FSE 2013.
- “Data Debugging... ” presented at ESEC/FSE NI 2013.
- Future of Software Engineering 2013 took place in July 2013.
- “Unifying FSM-Inference...” presented at ICSE 2013.
- “Entrusting Private Compu...” published in IEEE TDSC.
- “Understanding Regression...” presented at ICSE NIER 2013.
- “Speculative Analysis...” presented at OOPSLA 2012.
- “Proactive Detection of...” published in ESEC/FSE 2011 and is awarded an ACM SIGSOFT Distinguished Paper Award. Watch the talk.
I have previously worked with Prof. Leonard Adleman at the USC Laboratory for Molecular Science and with Prof. Michael D. Ernst at the MIT Computer Science and Artificial Intelligence Laboratory Program Analysis Group.
My research aims to improve how we build software systems, focusing on proving system correctness, mitigating bias, and enabling self-adaption and self-repair. I co-direct the LASER and PLASMA laboratories.
My lab's research focuses on high-risk, high-impact problems, with the aim of
fundamentally improving how engineers build systems. Sometimes we fail.
Sometimes we succeed and make a difference in industry, academia, or both. Our work has received a Microsoft Research Software Engineering Innovation Foundation Award, a Google Inclusion Research Award, an Amazon Research Award, a Google Faculty Research Award, and an NSF CAREER Award, as well as five ACM SIGSOFT and SIGPLAN Distinguished Paper Awards and a Best Paper Award, and has been funded by the NSF, DARPA, IARPA, Amazon, Dolby, Google, Kosa.ai, Microsoft, Meta, and Oracle. |
Automating formal verificationFormal verification—proving the correctness of software—is one of the most effective ways of improving software quality. But, despite significant help from interactive theorem provers and automation tools such as hammers, writing the required proofs is an incredibly difficult, time consuming, and error prone manual process. My work has pioneered ways to use natural language processing to synthesize formal verification proofs. Automating proof synthesis can drastically reduce costs of formal verification and improve software quality. The unique nature of formal verification allows us to combine multiple models to fully automatically prove 33.8% of formal verification theorems in Coq (ICSE'22 Distinguished Paper Award), and use large language models to synthesize guaranteed-correct proofs for 65.7% of mathematical theorems in Isabelle (ESEC/FSE'23 Distinguished Paper Award). |
Provably fair and safe software
|
Trust in machine learningIncreasingly, software systems use machine learning. Whether users understand how this technology functions can fundamentally affect their trust in these systems. We study how aspects of machine learning, such as precision and bias, affect people's perception and trust, and how explainability techniques can better educate users, engineers, and policymakers into making better, evidence-based decisions about machine learning. Our findings include that bias affects men and women differently when making trust-based decisions, and that visualization design choices have a significant impact on bias perception, and on the resulting trust. |
High-quality automated program repair
|
Proactive detection of collaboration conflicts
|
Privacy preservation in distributed computation
|
Automatically inferring precise models of system behaviorDebugging and improving systems requires understanding their behavior. Using source code and execution logs to understand behavior is a daunting task. We develop tools to aid understanding and development tasks, many of which involve automatically inferring a human-readable FSM-based behavioral model. This project is or has been funded by the NSF, Google, and Microsoft Research. We have shown that the inferred models can be used to detect software vulnerabilities (read) and are currently building model-based tools to improve test suite quality at Google. |
|
|
|
|
Reliability through smart redundancy
|
Self-adaptive systems
|
Bug cause analysisWhen regression tests break, can the information about the latest changes help find the cause of the failure? The cause might be a bug in recently added code, or it could instead be in old code that wasn't exercised in a fault-revealing way until now. Considering the minimal test-failing sets of recent changes, and maximal test-passing sets of those changes can identify surprises about the failure's cause. Read: Understanding Regression Failures through Test-Passing and Test-Failing Code Changes. |
DNA Self-Assembly
|
The fault-invariant classifier
|
Current PhD students (advisor):
|
|
|
|
|
Current PhD students (committee member):
|
|
|
|
|
Current Masters and undergraduate students:
- Marie Shvakel (undergraduate)
- Morgan Waterman (undergraduate)
- Simran Lekhwani (undergraduate)
Alumni postdocs:
|
|
|
Alumni PhD students (advisor):
|
|
|
|
|
Alumni PhD students (committee member):
|
|
|
|
|
|
|
|
|
Alumni Masters and undergraduate students:
- Declan Gray-Mullen received his Masters degree in 2022 and his BS degree in 2021 from UMass.
- Sarah Brockman received her Masters degree in 2021 and received her BS degree with honors in 2019 from UMass, currently an R&D Engineer at Kitware.
- Jennifer Halbleib received her Masters degree in 2020 from UMass, currently a Senior Data Scientist at Amazon Web Services.
- Donald Pinckney received his Masters degree in 2020 from UMass, currently getting his PhD at Northeastern University.
- Rico Angell received his Masters degree in 2019 and BS degree with honors in 2016 from UMass, currently getting his PhD at UMass.
- Tanya Asnani received her BS degree with honors in 2019 from UMass, currently at Bank of America Merrill Lynch.
- Jesse Bartola received his BS degree in 2019 from UMass, currently a senior software engineer at Hubspot.
- Aisiri Murulidhar received her BS degree in 2019 from UMass, currently a software engineer at Google, Inc.
- Natcha Simsiri received his Masters degree in 2018 and BS degree with honors in 2016 from UMass, currently at Lutron Electronics.
- Nicholas Perello received his BS degree with honors in 2018 from UMass, currently a PhD student at UMass.
- Ted Smith received his Masters degree in 2016 from UMass, currently at Bloomberg.
- Ryan Stanley received his BS degree with honors in 2016 from UMass, currently a software engineer at Amazon.com.
- Karthik Kannappan received a Masters degree in 2015 from UMass, now a software engineer at Juniper Networks.
- Armand Halbert received a Masters degree in 2015 from UMass, now a software engineer at IBM.
- Chris Ciollaro, received his BS degree in 2015 from UMass, currently a Masters student.
- Xiang Zhao (co-advised with Leon J. Osterweil) received a Masters degree in 2014 from UMass, now a software engineer at Google Inc.
- Nicholas Braga, honors thesis, received his BS degree in 2014 from UMass, currently a software engineer at BookBub.
- Brian Stapleton, received a BS degree in 2014 from UMass, now a software engineer at AdHarmonics Inc.
- Alice Ouyang, received a BS degree in 2013 from UW, now an IT Analyst at Liberty Mutual Insurance
- Brandon McNew (REU in summer of 2013), received a BS degree in 2013 from Hendrix College, now software developer at CUSi
- Jeanderson Barros (REU in summer of 2013)
- Roykrong Sukkerd, received a BS degree in 2013 from UW, now a PhD student at Carnegie Mellon University
- Haochen Wei, received a BS degree in 2013 from UW, now a software developer at LinkedIn
- Jonathan Ramaswamy, received a BS degree in 2012 from UW, now an associate consultant at Cirrus10
- Fall 2024: CS 320: Introduction to Software Engineering
- Fall 2024: CS 429: Software Engineering Project Management
- Fall 2024: CS 692P: Hot Topics in Software Engineering Research
- Spring 2024: CS 320: Introduction to Software Engineering
- Spring 2024: CS 429: Software Engineering Project Management
- Spring 2023: CS 621: Advanced Software Engineering: Analysis and Evaluation
- Fall 2022: CS 320: Introduction to Software Engineering
- Fall 2022: CS 429: Software Engineering Project Management
- Spring 2018: CS 692P: Hot Topics in Software Engineering Research
- Fall 2018: CS 520: Theory and Practice of Software Engineering
- Spring 2018: CS 621: Advanced Software Engineering: Analysis and Evaluation
- Spring 2018: CS 692P: Programming Languages & Systems Seminar
- Fall 2017: CS 520: Theory and Practice of Software Engineering
- Spring 2017: CS 521: Software Engineering: Analysis and Evaluation
- Spring 2017: CS 621: Advanced Software Engineering: Analysis and Evaluation
- Fall 2015: CS 521: Software Engineering: Analysis and Evaluation
- Fall 2015: CS 621: Advanced Software Engineering: Analysis and Evaluation
- Spring 2015: CS 320: Introduction to Software Engineering
- Spring 2015: CS H320: Introduction to Software Engineering Honors Colloquium
- Spring 2015: CS 529: Software Engineering Project Management
- Fall 2014: CS 521: Software Engineering: Analysis and Evaluation
- Fall 2014: CS 621: Advanced Software Engineering: Analysis and Evaluation
- Fall 2013: CS 521: Software Engineering: Analysis and Evaluation
- Fall 2013: CS 621: Advanced Software Engineering: Analysis and Evaluation
- Spring 2013: CS 320: Introduction to Software Engineering
- Spring 2013: CS H320: Introduction to Software Engineering Honors Colloquium
- Spring 2013: CS 529: Software Engineering Project Management
- Fall 2012: CS 521: Software Engineering: Analysis and Evaluation
- Fall 2012: CS 621: Advanced Software Engineering: Analysis and Evaluation
- Spring 2012: CSE590N: Software Engineering Seminar
- Winter 2012: CSE590N: Software Engineering Seminar
- Autumn 2011: CSE590N: Software Engineering Seminar
- Winter 2011: CSE403: Software Engineering
Involving undergraduates in research is central to my mission as faculty. I started my research career in earnest as an undergraduate and it is only due to the patience and support of my mentors that I am who I am today. I work hard to pay back the community by encouraging undergraduate students to experience research. But I don't consider this service; some of my best collaborations came from working with undergraduates. Focusing especially on groups underrepresented in our community, such as women and minorities, I have been lucky enough to work with brilliant undergraduate students, publishing top venue conference papers with them at FSE'11, ICSE'13, ASE'14, ISSRE'15, and IEEE TSE, as well as several shorter new ideas, emerging results, and demonstration papers at FSE'11 ICSE'13, ICSE'14, and ICSE'16. These students have gone on to great positions, both in industry and graduate school, pursuing their PhD degrees. For example, Roykrong Sukkerd (pictured on left at ICSE'13) is now a PhD student at Carnegie Mellon University, and Jenny Abrahamson received her Masters degree in Computer Science from the University of Washington and is currently a software engineer at Facebook Inc. Expanding my reach, through the NSF REU program, I have worked with several great students such as Brandon McNew from Hendrix College in Conway, Arkansas, USA, who would otherwise have no exposure to research. |
I attended public K–12 schools in Massachusetts and benefited
greatly from my education. At UMass, I am lucky to be surrounded by
opportunities to give back. I work with a local chapter of Girls Inc. to promote
science and technology to women aged 12–18 through the Eureka!
program. The women spend five weeks every summer at UMass
(returning for up to five years), working with faculty through
collaborative workshops to learn about STEM fields and have fun with
science! In the later years, students engage in one-on-one research
with faculty and get a great start on their college careers. Starting
in Fall 2015, Eureka! expanded to school-year Saturday workshops
and I am proud to be a part of that expansion. Watch one video about
Eureka!, and another one. |
My research strives to solve problems relevant to industry and the
real world. I collaborate with industry and my students often go on
internships to implement our work within companies. My work has been
supported by Microsoft (via a 2014 Microsoft Research SEIF award) and
Google (via a 2015 Google Faculty Research Award). |
Central to science, and to scientific careers, is the peer-review process. Unfortunately, research has shown overwhelming evidence that certain subconscious biases adversely affect the fairness of peer-review, most often hurting women and minorities. Fortunately, double-blind peer-review has been shown to successfully mitigate these negative affects. Regrettably, my research community, the Software Engineering research community, has been slow to employ double-blind reviewing at its top venues. Given the weight publications at these venues have in hiring and promotion decisions, I could not idly stand by. In late 2014, I began a campaign to convince the organizers of ICSE, the top conference in our field, to use double-blind review. In April 2015, I wrote an open letter to the community, laying out the research supporting the existence of bias in single-blind reviewing, and the benefits and potential costs of double-blind reviewing. ICSE took the proposal and evidence seriously, and I am currently serving on the ICSE Double Blind Review Task Force to concretely identify the needs, costs, and benefits of the process, as it applies directly to ICSE. ICSE 2018 will use the double blind-review process! Thanks to the hard work of my colleagues, several other Software Engineering conferences, including ASE, ISSTA, and FASE, have announced that they also will begin using double-blind reviewing. Read more about the scientific evidence for the need for double-blind reviewing. |
Organizing broadly inclusive, targeted-topic workshops is one of
the most effective ways to get students involved in research and to create
the kind of powerful collaborations that occur only when people with
real-world problems meet face to face with people who create
solutions. I have co-organized two such workshops. In 2013, I
co-organized the Future of
Software Engineering symposium (pictured on right) in Redmond, WA, USA to bring
together the most prominent Software Engineering researches to discuss
key future directions for the community. With generous support from the
NSF and Microsoft Research, 40 graduate and undergraduate students came
to learn about these directions, share their ideas, and reason about how
their research contributes to the greater good. Videos of the presentations
further increase the broader impact of this workshop. |
The Erdös number describes the "collaborative distance" between a person and the mathematician Paul Erdös, as measured by the authorship of scientific papers. Currently, my Erdös number is 3.
- Yuriy Brun coauthored with Leonard
M. Adleman:
DNA Triangles and Self-Assembled Hexagonal Tilings in the Journal of the American Chemical Society - Leonard M. Adleman coauthored with Andrew M.
Odlyzko:
Irreducibility testing and factorization of polynomials in the 1981 Symposium on the Foundations of Computer Science - Andrew M. Odlyzko coauthored with Paul
Erdös:
On the density of odd integers of the form (p − 1)2−n and related questions in the Journal of Number Theory
The world of academia is one of the last professions that follows the master-apprentice relationship to indoctrinate new members. In order to become a doctor of philosophy, a crucial step in the quest to become a professor and a researcher, each student is closely and personally advised by his or her mentor. That mentor, often referred to as one's academic "father" or "mother," is responsible for shaping young minds into those of diligent, honest, and ethical scientists. Below is the genealogy of my upbringing, in chronological order, tracing back to the mid 17th century, and including the founders of calculus, basic mathematical theory, and computer science.
- Erhard Weigel (who received his Ph.D. in 1650) spent much of his life popularizing science to make it more accessible to the public, and now has the Weigel crater on the moon named after him.
- His student, Gottfried Wilhelm Leibniz (1666), invented calculus, discovered the binary system and the foundation of virtually all modern computer architectures.
- Gottfried's student, Jacob Bernoulli (1684), was the first to develop the technique for solving separable differential equations and introduced the law of large numbers.
- His brother and student, Johann Bernoulli (1694), supported Descartes' vortex theory over Newton's theory of gravitation, which ultimately delayed acceptance of Newton's theory in Europe. Perhaps in a display of karma, Johann lost the naming rights to the L'Hôpital's rule, despite inventing it.
- While young, Johann educated Leonhard Euler (1726), the preeminent mathematician of the 18th century, who introduced much of modern terminology and notation to mathematics, particularly analysis, including the notion of a mathematical function. He has been featured on Swiss bank notes and numerous postage stamps has had asteroid named after him.
- Euler advised Joseph Louis Lagrange, who is most famous for inventing the Lagrange multipliers for finding the extrema of functions taking into account possible constraints.
- One of his three students, Simeon Denis Poisson (1802) is best known for the Poisson's equation, a generalization of Laplace's equation, and also for the Poisson distribution.
- Simeon taught Michel Chasles (1814), who established several important theorems (all called Chasles' theorems), one of which, on solid body kinematics, was seminal for understanding solid bodies' motions and to the development of the theories of dynamics of rigid bodies.
- Michel's student, Hubert Anson Newton (1850), was a worldwide authority on the subjects of meteors and comets, and educated Eliakim Hastings Moore.
- Eliakim Hastings Moore (1885) proved that every finite field is a Galois field, reformulated Hilbert's axioms for geometry so that points were the only primitive notion, and showed that one of Hilbert's axioms for geometry was redundant.
- One of Eliakim's students, Oswald Veblen (1903), was involved with the project that produced the pioneering ENIAC electronic digital computer.
- His student, Alonzo Church (1927), was the author of λ-calculus and the hypothesis, known as Church's Thesis, that every effectively calculable function (effectively decidable predicate) is general recursive. Together with Turing's thesis, Church's thesis forms the foundation of computer science as we know it.
- His student, John Barkley Rosser (1934), showed that the original λ-calculus was inconsistent.
- John's student, Gerald Enoch Sacks (1961), and, in turn, his student, James Claggett Owings, Jr. (1966), worked on recursion theory.
- James' student, Leon J. Osterweil (1971), works in the field of software engineering, concentrating on specification and analysis of the processes that guide software development.
- Leon's student, Richard N. Taylor (1980), founded the Department of Informatics at the University of California Irvine, is the director of the Institute for Software Research, and works on software design and architectures, especially event-based and peer-to-peer systems.
- Richard's student, and my advisor, Nenad Medvidović (1999), is the director of the USC Center for Systems and Software Engineering and his work focuses on architecture-based software development.
- I, Yuriy Brun (2008), one of Nenad's students, am working on easing the process of developing software by automating certain aspects of ensuring robustness, security, and scalability in software systems.