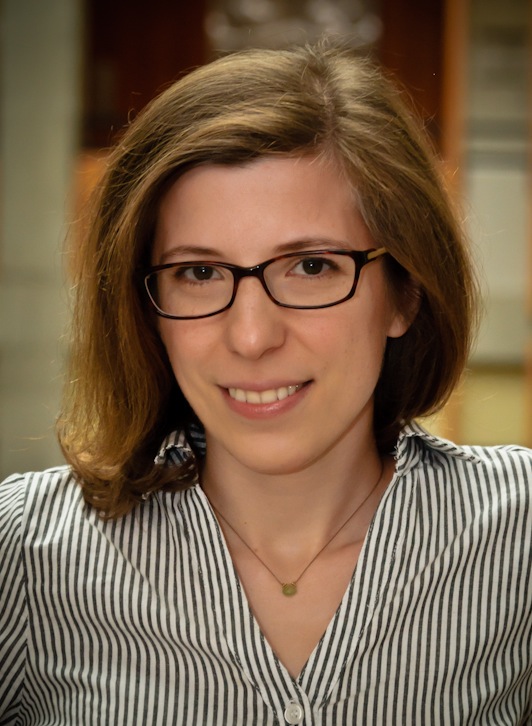
Alexandra Meliou
Associate Professor
Associate Chair for
Faculty Development
Robert and Donna Manning
College of Information
and Computer Sciences
140 Governors Drive
University of Massachusetts
Amherst, MA 01003-9264 USA
Email: | |
Office: | 330 |
Phone: | +1-413-545-3788 |
Fax: | +1-413-545-1249 |
Research
DREAM lab: Data-systems Research for Exploration, Analytics, and Mining
I co-direct the DREAM lab, with an awesome group of people! UMass is a vibrant place, and one of the top universities in the US for data management research!
My interests
Data is critical in almost every aspect of society, including education, technology, healthcare, economy, and science. Poor understanding and handling of data, data biases, poor data quality, and errors in data-driven processes are detrimental in all domains that rely on data. My research augments data management with user-facing functionality that helps people make sense of their data and use it effectively, at a time when data becomes increasingly unpredictable, unwieldy, and unmanageable. I focus on issues of provenance, causality, explanations, data quality, usability, and data and algorithmic bias.
Usability and analysis
As data is now a staple in so many aspects of human activity, the audience for data technologies has expanded to include a varied range of users: from non-experts wishing to peruse datasets, to domain experts with specialized data processing needs. Data systems have not adapted to address these demands effectively: databases' specialized query languages and structure create barriers for non-experts, while the lack of native support for important computing needs leaves experts to develop application-specific solutions themselves. Our work removes data-use barriers by simplifying access for non-experts to data and by augmenting database functionality with advanced problem-solving capabilities, thus simplifying analytics workflows by moving them closer to the data.
![]() |
PackageBuilder: supporting queries for packages [Project page]
Traditional database queries follow a simple model: they define constraints that each tuple in the result must satisfy. This model is computationally efficient, as the database system can evaluate the query conditions on each tuple individually. However, many practical, real-world problems require a collection of result tuples—which we call a package—to satisfy constraints collectively, rather than individually. We developed an end-to-end system that supports package queries, allowing the declarative specification and efficient evaluation of a significant class of constrained optimization problems within a database. Publications: [PVLDB 2016] [SIGMOD Record 2017] [VLDBJ 2017] [CACM 2018] ![]() |
![]() |
SQuID: Semantic-similarity-aware Query Intent Discovery [Project page]
Non-experts cannot easily peruse relational data, as traditional query interfaces allow data retrieval through well-structured queries. To write such queries, one needs expertise in the query language (typically SQL) and knowledge of the potentially complex database schema. Unfortunately, non-expert users typically lack both. SQuID infers query intent effectively by leveraging the data in the database to understand the context of the provided examples. SQuID's abduction-aware probabilistic model captures esoteric and complex semantic contexts, outperforming the state of the art. Publications: [PVLDB 2019][SIGMOD 2018 demo] |
Fairness and diversity
Data-driven software has the ability to shape human behavior: it affects the products we view and purchase, the news articles we read, the social interactions we engage in, and, ultimately, the opinions we form. Yet, data is an imperfect medium, tainted by errors, omissions, and biases. As a result, discrimination shows up in many data-driven applications, such as advertisements, hotel bookings, image search, and vendor services. Biases in data and software risk forming, propagating, and perpetuating biases in society. Data management research should develop tools to detect, inform, and mitigate the effects of bias, skew, and misuse in data-driven processes.
![]() |
Fairness testing [Project page]
Our work studied software fairness and discrimination and produced a testing-based method for measuring if and how much software discriminates, focusing on causality in discriminatory behavior. Our approach, Themis, is the first framework of its kind that automatically generates efficient test suites to measure discrimination. Our techniques rely on reasoning about causal relationships between inputs and outputs of a system. Understanding how inputs affect software behavior can empower developers to control for bias in data and ensure more fair use of software systems. Publications: [ESEC/FSE 2017] [ESEC/FSE 2018 demo] [ESEC/FSE 2018 vision] ![]() |
![]() |
Fast diverse data retrieval
Data skew is often a cause of algorithmic bias, and the ability to retrieve balanced, diverse datasets can mitigate the underlying problem. Diversification is one common way to present representative results to users, and it is employed by many real-world systems. However, providing diverse results for general range queries (i.e., queries that return a subset of the data based on filtering conditions) efficiently and scalably remains challenging. Our work introduces a general, index-based algorithm for diversifying the results of multi-dimensional range queries over a single relation. At a high level, our algorithm transforms each range query into a set of subordinate searches, performs these searches using a novel index structure, the RC-Index. Publications: [PVLDB 2018] |
Data quality
Data quality has long been a focus of data management research, but our data quality challenges have only grown. Data is produced at unprecedented rates, from sources that are broad, varied, and unreliable, and through large-scale processes that introduce their own inaccuracies (e.g., structured data extraction from unstructured text). Traditional data cleaning techniques identify discrepancies and purge datasets of errors, but they treat the manifestation of a problem, not its root cause. They disregard the fact that errors are often systemic, inherent to the process that produces the data, and thus will keep occurring unless the problems are corrected at their source. Our work offers crucial insights into data quality issues: instead of repairing the errors themselves, our research focuses on diagnosing the reasons for the errors and identifying repairs in the processes that produce the data.
![]() |
Data X-Ray: Diagnosing errors in data systems Data X-Ray is a diagnostic framework for profiling errors in data and determining systemic reasons for them in internet-scale knowledge extraction pipelines. This setting is challenging due to the large scale of the data, the prevalence of errors, and the complexity of the system. Publications: [SIGMOD 2015] [PVLDB 2015 demo] |
![]() |
QFix: Diagnosing errors in relational logs Relational databases are often dynamic, and even when data is cleaned, new errors can be introduced by applications and users who interact with the data. Subsequent valid updates can obscure these errors and propagate them through the dataset causing more discrepancies. Any discovered errors tend to be corrected superficially, on a case-by-case basis, further obscuring the true underlying cause, and making detection of the remaining errors harder. QFix derives explanations and repairs for discrepancies in relational data by analyzing the effects of queries that operated on the data and identifying potential mistakes in those queries. Publications: [SIGMOD 2017] [SIGMOD 2016 demo] |
Causality and explanations
Today's data is vast and often unreliable and the systems that process data are increasingly complex. Even simple transformations through database queries obscure the origins of data and the derivation of results. The goal of my research is to promote users' trust in data and systems through support for understanding and explanations. Explanations provide opportunities for systems to interact with humans and obtain feedback, improving their operation. Explanations also allow domain experts and system developers to understand system decisions and improve system function.
![]() |
Causal analysis and explanations in data management [Project page (causality)]
Our research investigates techniques that help users understand the results of their queries by analyzing the history of data transformations (provenance). Unfortunately, using the provenance to explain query results is often impractical, as provenance information can grow very large even for simple transformations and modest-size datasets. Our work refines provenance information by analyzing the causal contributions of data to a result, and develops explanation frameworks for a variety of data-driven settings. Publications (sample): [PVLDB 2019] [DE Bulletin 2018] [EDBT 2017] [PVLDB 2015] [PVLDB 2014 tutorial] |
Publications
2024 | |
[ICDE] | Ke Yang and Alexandra Meliou, Non-Invasive Fairness in Learning through the Lens of Data Drift, in 40th IEEE International Conference on Data Engineering, 2024. [details] [pdf] |
[PVLDB] | Anh L. Mai, Pengyu Wang, Azza Abouzied, Matteo Brucato, Peter J. Haas, and Alexandra Meliou, Scaling Package Queries to a Billion Tuples via Hierarchical Partitioning and Customized Optimization, PVLDB, vol. 17, 2024. [details] [pdf] |
2023 | |
[SIGMOD Record] | Alexandra Meliou, Sourav S. Bhowmick, Karl Aberer, Divy Agrawal, Angela Bonifati, Vanessa Braganholo, Floris Geerts, Wolfgang Lehner, and Divesh Srivastava, Peer-Reviewing Processes and Incentives: Data Management Community Survey Results, SIGMOD Rec., vol. 52, no. 4, 2023. [details] [pdf] |
[SIGMOD Record] | Sihem Amer-Yahia, Divyakant Agrawal, Yael Amsterdamer, Sourav S. Bhowmick, Angela Bonifati, Renata Borovica-Gajic, Jesús Camacho-Rodr\'ıguez, Barbara Catania, Panos K. Chrysanthis, Carlo Curino, Jérôme Darmont, Gillian Dobbie, Amr El Abbadi, Avrilia Floratou, Juliana Freire, Alekh Jindal, Vana Kalogeraki, Sujaya Maiyya, Alexandra Meliou, Madhulika Mohanty, Behrooz Omidvar-Tehrani, Fatma Özcan, Liat Peterfreund, Wenny Rahayu, Shazia Sadiq, Sana Sellami, Utku Sirin, Wang-Chiew Tan, Bhavani Thuraisingham, Yuanyuan Tian, Pinar Tözün, Genoveva Vargas-Solar, Neeraja J. Yadwadkar, Victor Zakhary, and Meihui Zhang, Diversity, Equity and Inclusion Activities in Database Conferences: A 2022 Report, SIGMOD Rec., vol. 52, no. 2, 2023, pp. 38–42. [details] [pdf] [url] [doi] |
2022 | |
[DEBul] | Azza Abouzied, Peter J. Haas, and Alexandra Meliou, In-Database Decision Support: Opportunities and Challenges, IEEE Data Engineering Bulletin, vol. 45, no. 3, sep 2022, pp. 102–115. [details] [pdf] |
[SIGMOD] | Maliha Tashfia Islam, Anna Fariha, Alexandra Meliou, and Babak Salimi, Through the Data Management Lens: Experimental Analysis and Evaluation of Fair Classification, in SIGMOD '22: International Conference on Management of Data, ACM, pp. 232–246. [details] [pdf] [url] [doi] |
[SIGMOD] | Sainyam Galhotra, Anna Fariha, Raoni Lourenço, Juliana Freire, Alexandra Meliou, and Divesh Srivastava, DataPrism: Exposing Disconnect between Data and Systems, in SIGMOD '22: International Conference on Management of Data, ACM, pp. 217–231. [details] [pdf] [url] [doi] |
[SIGMOD Record] | Sihem Amer-Yahia, Yael Amsterdamer, Sourav S. Bhowmick, Angela Bonifati, Philippe Bonnet, Renata Borovica-Gajic, Barbara Catania, Tania Cerquitelli, Silvia Chiusano, Panos K. Chrysanthis, Carlo Curino, Jérôme Darmont, Amr El Abbadi, Avrilia Floratou, Juliana Freire, Alekh Jindal, Vana Kalogeraki, Georgia Koutrika, Arun Kumar, Sujaya Maiyya, Alexandra Meliou, Madhulika Mohanty, Felix Naumann, Nele Sina Noack, Fatma Özcan, Liat Peterfreund, Wenny Rahayu, Wang-Chiew Tan, Yuanyuan Tian, Pinar Tözün, Genoveva Vargas-Solar, Neeraja J. Yadwadkar, and Meihui Zhang, Diversity and Inclusion Activities in Database Conferences: A 2021 Report, SIGMOD Record, vol. 51, no. 2, 2022, pp. 69–73. [details] [pdf] [url] [doi] |
[ICDT] | Raghavendra Addanki, Andrew McGregor, Alexandra Meliou, and Zafeiria Moumoulidou, Improved Approximation and Scalability for Fair Max-Min Diversification, in 25th International Conference on Database Theory (ICDT), Schloss Dagstuhl - Leibniz-Zentrum für Informatik, 2022, pp. 7:1–7:21. [details] [pdf] [url] [doi] |
2021 | |
[NewSum] | Nishant Yadav, Matteo Brucato, Anna Fariha, Oscar Yongquist, Julian Killingback, Alexandra Meliou, and Peter J. Haas, SubSumE: A Dataset for Subjective Summary Extraction from Wikipedia Documents, in New Frontiers in Summarization workshop (at EMNLP 2021). [details] [pdf] |
[SIGMOD] | Anna Fariha, Ashish Tiwari, Alexandra Meliou, Arjun Radhakrishna, and Sumit Gulwani, CoCo: Interactive Exploration of Conformance Constraints for Data Understanding and Data Cleaning, in Proceedings of the ACM SIGMOD International Conference on Management of Data (SIGMOD), 2021 (Demonstration paper). [details] [pdf] [doi] |
[SIGMOD] | Anna Fariha, Ashish Tiwari, Alexandra Meliou, Arjun Radhakrishna, and Sumit Gulwani, Conformance Constraint Discovery: Measuring Trust in Data-Driven Systems, in Proceedings of the ACM SIGMOD International Conference on Management of Data (SIGMOD), 2021 ([ACM SIGMOD Comprehensive Reproducibility Award]). [details] [pdf] [doi] |
[ICDT] | Zafeiria Moumoulidou, Andrew McGregor, and Alexandra Meliou, Diverse Data Selection under Fairness Constraints, in International Conference on Database Theory, (ICDT), 2021, pp. 11:1–11:25. [details] [pdf] |
[FnT2021] | Boris Glavic, Alexandra Meliou, and Sudeepa Roy, Trends in Explanations: Understanding and Debugging Data-driven Systems, Foundations and TrendsĀ® in Databases, vol. 11, no. 3, 2021, pp. 226–318. [details] [pdf] [url] [doi] |
2020 | |
[ICSE] | Brittany Johnson, Yuriy Brun, and Alexandra Meliou, Causal Testing: Understanding Defects' Root Causes, in Proceedings of the 42nd International Conference on Software Engineering (ICSE), 2020 ([ACM SIGSOFT Distinguished Artifact Award]). [details] [pdf] [doi] |
[PODS] | Cibele Freire, Wolfgang Gatterbauer, Neil Immerman, and Alexandra Meliou, New Results for the Complexity of Resilience for Binary Conjunctive Queries with Self-Joins, in Symposium on Principles of Database Systems (PODS), 2020, pp. 271–284. [details] [pdf] [doi] |
[SIGMOD] | Anna Fariha, Suman Nath, and Alexandra Meliou, Causality-Guided Adaptive Interventional Debugging, in Proceedings of the ACM SIGMOD International Conference on Management of Data (SIGMOD), 2020, pp. 431–446. [details] [pdf] [doi] |
[PVLDB] | Anna Fariha, Matteo Brucato, Peter J. Haas, and Alexandra Meliou, SuDocu: Summarizing Documents by Example, PVLDB, vol. 13, no. 12, 2020, pp. 2861ā2864 (Demonstration paper [Best Demonstration Runner Up Award]). [details] [pdf] |
[PVLDB] | Matteo Brucato, Miro Mannino, Azza Abouzied, Peter J. Haas, and Alexandra Meliou, sPaQLTooLs: A Stochastic Package Query Interface for Scalable Constrained Optimization, PVLDB, vol. 13, no. 12, 2020, pp. 2881ā2884 (Demonstration paper [Best Demonstration Award]). [details] [pdf] |
[SIGMOD] | Matteo Brucato, Nishant Yadav, Azza Abouzied, Peter Haas, and Alexandra Meliou, Stochastic Package Queries in Probabilistic Databases, in Proceedings of the ACM SIGMOD International Conference on Management of Data (SIGMOD), 2020, pp. 269–283. [details] [pdf] [doi] |
2019 | |
[ICDE] | Xiaolan Wang, Luna Dong, Yang Li, and Alexandra Meliou, MIDAS: Finding the Right Web Sources to Fill Knowledge Gaps, in 35th IEEE International Conference on Data Engineering, 2019, pp. 578–589. [details] [pdf] |
[CACM] | Matteo Brucato, Azza Abouzied, and Alexandra Meliou, Scalable Computation of High-Order Optimization Queries, Communications of the ACM, vol. 62, no. 2, feb 2019, pp. 108–116 ([Research Highlight]). [details] [pdf] [url] [doi] |
[PVLDB] | Xiaolan Wang and Alexandra Meliou, Explain3D: Explaining Disagreements in Disjoint Datasets, PVLDB, vol. 12, no. 7, 2019, pp. 779–792. [details] [pdf] |
[PVLDB] | Anna Fariha and Alexandra Meliou, Example-Driven Query Intent Discovery: Abductive Reasoning using Semantic Similarity, PVLDB, vol. 12, no. 11, 2019, pp. 1262–1275. [details] [pdf] |
2018 | |
[ESEC/FSE] | Yuriy Brun and Alexandra Meliou, Software Fairness, in Proceedings of the New Ideas and Emerging Results Track at the 26th ACM Joint European Software Engineering Conference and Symposium on the Foundations of Software Engineering (ESEC/FSE), 2018, pp. 754–759. [details] [pdf] [doi] |
[ESEC/FSE] | Rico Angell, Brittany Johnson, Yuriy Brun, and Alexandra Meliou, Themis: Automatically Testing Software for Discrimination, in Proceedings of the Demonstrations Track at the The 26th ACM Joint European Software Engineering Conference and Symposium on the Foundations of Software Engineering (ESEC/FSE), 2018, pp. 871–875 (Demonstration paper). [details] [pdf] [doi] |
[PVLDB] | Yue Wang, Alexandra Meliou, and Gerome Miklau, RC-Index: Diversifying Answers to Range Queries, PVLDB, vol. 11, no. 7, sep 2018, pp. 773–786. [details] [pdf] [doi] |
[DEBul] | Xiaolan Wang, Laura Haas, and Alexandra Meliou, Explaining Data Integration, IEEE Data Engineering Bulletin, vol. 41, no. 2, jun 2018, pp. 47–58. [details] [pdf] |
[SIGMOD] | Anna Fariha, Sheikh Muhammad Sarwar, and Alexandra Meliou, SQuID: Semantic Similarity-Aware Query Intent Discovery, in Proceedings of the ACM SIGMOD International Conference on Management of Data (SIGMOD), 2018, pp. 1745–1748 (Demonstration paper). [details] [pdf] [doi] |
[VLDBJ] | Matteo Brucato, Azza Abouzied, and Alexandra Meliou, Package queries: efficient and scalable computation of high-order constraints, The VLDB Journal, vol. 27, no. 1, 2018 (Special Issue on Best Papers of VLDB 2016). [details] [pdf] [doi] |
2017 | |
[ESEC/FSE] | Sainyam Galhotra, Yuriy Brun, and Alexandra Meliou, Fairness Testing: Testing Software for Discrimination, in Proceedings of 2017 11th Joint Meeting of the European Software Engineering Conference and the ACM SIGSOFT Symposium on the Foundations of Software Engineering, pp. 498–510 ([ACM SIGSOFT Distinguished Paper Award]). [details] [pdf] [doi] |
[SIGMOD] | Xiaolan Wang, Alexandra Meliou, and Eugene Wu, QFix: Diagnosing errors through query histories, in Proceedings of the ACM SIGMOD International Conference on Management of Data (SIGMOD), 2017, pp. 1369–1384. [details] [pdf] [doi] |
[EDBT] | Haopeng Zhang, Yanlei Diao, and Alexandra Meliou, EXStream: Explaining Anomalies in Event Stream Monitoring, in 20th International Conference on Extending Database Technology (EDBT), 2017, pp. 156–167. [details] [pdf] [doi] |
[SIGMOD Record] | Matteo Brucato, Azza Abouzied, and Alexandra Meliou, A Scalable Execution Engine for Package Queries, SIGMOD Record, vol. 46, no. 1, mar 2017, pp. 24–31 ([ACM SIGMOD Research Highlight Award]). [details] [pdf] [doi] |
2016 | |
[SIGMOD] | Xiaolan Wang, Alexandra Meliou, and Eugene Wu, QFix: Demonstrating error diagnosis in query histories, in Proceedings of the ACM SIGMOD International Conference on Management of Data (SIGMOD), 2016, pp. 2177–2180 (Demonstration paper). [details] [pdf] [doi] |
[PVLDB] | Yue Wang, Alexandra Meliou, and Gerome Miklau, Lifting the Haze off the Cloud: A Consumer-Centric Market for Database Computation in the Cloud, PVLDB, vol. 10, no. 4, 2016, pp. 373–384. [details] [pdf] [doi] |
[PVLDB] | Matteo Brucato, Juan Felipe Beltran, Azza Abouzied, and Alexandra Meliou, Scalable Package Queries in Relational Database Systems, PVLDB, vol. 9, no. 7, 2016, pp. 575–587 ([Best papers of VLDB 2016]). [details] [pdf] [doi] |
2015 | |
[ISSTA] | Kıvanç Muşlu, Yuriy Brun, and Alexandra Meliou, Preventing Data Errors with Continuous Testing, in Proceedings of the ACM SIGSOFT International Symposium on Software Testing and Analysis (ISSTA), 2015, pp. 373–384. [details] [pdf] [doi] |
[PVLDB] | Xiaolan Wang, Mary Feng, Yue Wang, Luna Dong, and Alexandra Meliou, Error Diagnosis and Data Profiling with Data X-Ray, PVLDB, vol. 8, no. 12, 2015, pp. 1984–1987 (Demonstration paper). [details] [pdf] [doi] |
[SIGMOD] | Xiaolan Wang, Xin Luna Dong, and Alexandra Meliou, Data X-Ray: A Diagnostic Tool for Data Errors, in Proceedings of the ACM SIGMOD International Conference on Management of Data (SIGMOD), 2015, pp. 1231–1245. [details] [pdf] [doi] |
[PVLDB] | Cibele Freire, Wolfgang Gatterbauer, Neil Immerman, and Alexandra Meliou, A Characterization of the Complexity of Resilience and Responsibility for Self-join-free Conjunctive Queries, PVLDB, vol. 9, no. 3, 2015, pp. 180–191. [details] [pdf] [doi] |
2014 | |
[SIGMOD] | Ravali Pochampally, Anish Das Sarma, Xin Luna Dong, Alexandra Meliou, and Divesh Srivastava, Fusing Data with Correlations, in Proceedings of the ACM SIGMOD International Conference on Management of Data (SIGMOD), 2014, pp. 433–444. [details] [pdf] [doi] |
[PVLDB] | Alexandra Meliou, Sudeepa Roy, and Dan Suciu, Causality and Explanations in Databases, PVLDB, vol. 7, no. 13, 2014, pp. 1715–1716 (Tutorial). [details] [pdf] [doi] |
[SIGMOD] | Kevin Fernandes, Matteo Brucato, Rahul Ramakrishna, Azza Abouzied, and Alexandra Meliou, PackageBuilder: querying for packages of tuples, in Proceedings of the ACM SIGMOD International Conference on Management of Data (SIGMOD), 2014, pp. 1613–1614 (Undergraduate research poster). [details] [pdf] [doi] |
[PVLDB] | Matteo Brucato, Rahul Ramakrishna, Azza Abouzied, and Alexandra Meliou, PackageBuilder: From Tuples to Packages, PVLDB, vol. 7, no. 13, 2014, pp. 1593–1596 (Demonstration paper). [details] [pdf] [doi] |
[DATA4U] | Matteo Brucato, Azza Abouzied, and Alexandra Meliou, Improving Package Recommendations Through Query Relaxation, in Proceedings of the 1st International DATA4U Workshop, in conjunction with VLDB, 2014. [details] [pdf] [doi] |
2013 | |
[ESEC/FSE] | Kıvanç Muşlu, Yuriy Brun, and Alexandra Meliou, Data Debugging with Continuous Testing, in Proceedings of the New Ideas Track at the 9th Joint Meeting of the European Software Engineering Conference and ACM SIGSOFT Symposium on the Foundations of Software Engineering (ESEC/FSE13), 2013, pp. 631–634. [details] [pdf] [doi] |
2012 | |
[SIGMOD] | Alexandra Meliou, Yisong Song, and Dan Suciu, Tiresias: A Demonstration of How-To Queries, in Proceedings of the ACM SIGMOD International Conference on Management of Data (SIGMOD), 2012, pp. 709–712 (Demonstration paper [Best Demonstration Award]). [details] [pdf] [doi] |
[SIGMOD] | Alexandra Meliou and Dan Suciu, Tiresias: The Database Oracle for How-To Queries, in Proceedings of the ACM SIGMOD International Conference on Management of Data (SIGMOD), 2012, pp. 337–348. [details] [pdf] [doi] |
2011 | |
[SIGMOD] | Alexandra Meliou, Wolfgang Gatterbauer, Suman Nath, and Dan Suciu, Tracing Data Errors with View-Conditioned Causality, in Proceedings of the ACM SIGMOD International Conference on Management of Data (SIGMOD), 2011, pp. 505–516. [details] [pdf] [doi] |
[TaPP] | Alexandra Meliou, Wolfgang Gatterbauer, and Dan Suciu, Bringing Provenance to its Full Potential Using Causal Reasoning, in 3rd USENIX Workshop on the Theory and Practice of Provenance (TaPP), 2011. [details] [pdf] |
[TaPP] | Wolfgang Gatterbauer, Alexandra Meliou, and Dan Suciu, Default-all is Dangerous!, in 3rd USENIX Workshop on the Theory and Practice of Provenance (TaPP), 2011. [details] [pdf] |
[PVLDB] | Alexandra Meliou, Wolfgang Gatterbauer, and Dan Suciu, Reverse Data Management, PVLDB, vol. 4, no. 11, 2011, pp. 1490–1493. [details] [pdf] |
2010 | |
[NIPS] | Abhay Jha, Vibhav Gogate, Alexandra Meliou, and Dan Suciu, Lifted Inference Seen from the Other Side: The Tractable Features, in 24th Annual Conference on Neural Information Processing Systems (NIPS), 2010, pp. 973–981. [details] [pdf] |
[MUD] | Alexandra Meliou, Wolfgang Gatterbauer, Katherine F. Moore, and Dan Suciu, Why So? or Why No? Functional Causality for Explaining Query Answers, in Proceedings of the 4th International VLDB workshop on Management of Uncertain Data (MUD) in conjunction with VLDB, 2010, pp. 3–17. [details] [pdf] |
[AMW] | Alexandra Meliou, Carlos Guestrin, and Joseph M. Hellerstein, Multiresolution Cube Estimators for Sensor Network Aggregate Queries, in Proceedings of the 4th Alberto Mendelzon International Workshop on Foundations of Data Management (AMW), 2010. [details] [pdf] |
[PVLDB] | Alexandra Meliou, Wolfgang Gatterbauer, Katherine F. Moore, and Dan Suciu, The Complexity of Causality and Responsibility for Query Answers and non-Answers, PVLDB, vol. 4, no. 1, 2010, pp. 34–45. [details] [pdf] |
[DEBul] | Alexandra Meliou, Wolfgang Gatterbauer, Joseph Y. Halpern, Christoph Koch, Katherine F. Moore, and Dan Suciu, Causality in Databases, IEEE Data Engineering Bulletin, vol. 33, no. 3, 2010, pp. 59–67. [details] [pdf] |
2009 | |
[PhD] | Alexandra Meliou, Querying Uncertain Data in Resource Constrained Settings, Ph.D. dissertation, University of California, Berkeley, Berkeley, CA, 2009. [details] [pdf] |
[IPSN] | Alexandra Meliou, Carlos Guestrin, and Joseph M. Hellerstein, Approximating Sensor Network Queries using In-Network Summaries, in Proceedings of the 8th International Conference on Information Processing in Sensor Networks (IPSN), 2009, pp. 229–240. [details] [pdf] |
2008 | |
[InfSci] | Theodore Dalamagas, Alexandra Meliou, and Timos K. Sellis, Modeling and Manipulating the Structure of Hierarchical Schemas for the Web, Information Sciences, Elsevier, vol. 178, no. 4, 2008, pp. 985–1010. [details] [pdf] [doi] |
2007 | |
[AAAI] | Alexandra Meliou, Andreas Krause, Carlos Guestrin, and Joseph M. Hellerstein, Nonmyopic Informative Path Planning in Spatio-Temporal Models, in Proceedings of the 22nd National Conference on Artificial Intelligence (AAAI), 2007, pp. 602–607. [details] [pdf] |
2006 | |
[IPSN] | Alexandra Meliou, David Chu, Joseph M. Hellerstein, Carlos Guestrin, and Wei Hong, Data Gathering Tours in Sensor Networks, in Proceedings of the 5th International Conference on Information Processing in Sensor Networks (IPSN), 2006, pp. 43–50. [details] [pdf] [doi] |
2005 | |
[MSc] | Alexandra Meliou, Data Gathering Tours in Sensor Networks, Master's thesis, University of California, Berkeley, Berkeley, CA, 2005. [details] [pdf] |
2003 | |
[BSc] | Alexandra Meliou, Modeling and Exploring the Algebraic Properties of Hierarchical Structures, Athens, Greece, 2003. [details] [pdf] |
[PVLDB] | Anh L. Mai, Pengyu Wang, Azza Abouzied, Matteo Brucato, Peter J. Haas, and Alexandra Meliou, Scaling Package Queries to a Billion Tuples via Hierarchical Partitioning and Customized Optimization, PVLDB, vol. 17, 2024. [details] [pdf] |
[SIGMOD Record] | Alexandra Meliou, Sourav S. Bhowmick, Karl Aberer, Divy Agrawal, Angela Bonifati, Vanessa Braganholo, Floris Geerts, Wolfgang Lehner, and Divesh Srivastava, Peer-Reviewing Processes and Incentives: Data Management Community Survey Results, SIGMOD Rec., vol. 52, no. 4, 2023. [details] [pdf] |
[SIGMOD Record] | Sihem Amer-Yahia, Divyakant Agrawal, Yael Amsterdamer, Sourav S. Bhowmick, Angela Bonifati, Renata Borovica-Gajic, Jesús Camacho-Rodr\'ıguez, Barbara Catania, Panos K. Chrysanthis, Carlo Curino, Jérôme Darmont, Gillian Dobbie, Amr El Abbadi, Avrilia Floratou, Juliana Freire, Alekh Jindal, Vana Kalogeraki, Sujaya Maiyya, Alexandra Meliou, Madhulika Mohanty, Behrooz Omidvar-Tehrani, Fatma Özcan, Liat Peterfreund, Wenny Rahayu, Shazia Sadiq, Sana Sellami, Utku Sirin, Wang-Chiew Tan, Bhavani Thuraisingham, Yuanyuan Tian, Pinar Tözün, Genoveva Vargas-Solar, Neeraja J. Yadwadkar, Victor Zakhary, and Meihui Zhang, Diversity, Equity and Inclusion Activities in Database Conferences: A 2022 Report, SIGMOD Rec., vol. 52, no. 2, 2023, pp. 38–42. [details] [pdf] [url] [doi] |
[SIGMOD Record] | Sihem Amer-Yahia, Yael Amsterdamer, Sourav S. Bhowmick, Angela Bonifati, Philippe Bonnet, Renata Borovica-Gajic, Barbara Catania, Tania Cerquitelli, Silvia Chiusano, Panos K. Chrysanthis, Carlo Curino, Jérôme Darmont, Amr El Abbadi, Avrilia Floratou, Juliana Freire, Alekh Jindal, Vana Kalogeraki, Georgia Koutrika, Arun Kumar, Sujaya Maiyya, Alexandra Meliou, Madhulika Mohanty, Felix Naumann, Nele Sina Noack, Fatma Özcan, Liat Peterfreund, Wenny Rahayu, Wang-Chiew Tan, Yuanyuan Tian, Pinar Tözün, Genoveva Vargas-Solar, Neeraja J. Yadwadkar, and Meihui Zhang, Diversity and Inclusion Activities in Database Conferences: A 2021 Report, SIGMOD Record, vol. 51, no. 2, 2022, pp. 69–73. [details] [pdf] [url] [doi] |
[DEBul] | Azza Abouzied, Peter J. Haas, and Alexandra Meliou, In-Database Decision Support: Opportunities and Challenges, IEEE Data Engineering Bulletin, vol. 45, no. 3, sep 2022, pp. 102–115. [details] [pdf] |
[FnT2021] | Boris Glavic, Alexandra Meliou, and Sudeepa Roy, Trends in Explanations: Understanding and Debugging Data-driven Systems, Foundations and TrendsĀ® in Databases, vol. 11, no. 3, 2021, pp. 226–318. [details] [pdf] [url] [doi] |
[PVLDB] | Anna Fariha, Matteo Brucato, Peter J. Haas, and Alexandra Meliou, SuDocu: Summarizing Documents by Example, PVLDB, vol. 13, no. 12, 2020, pp. 2861ā2864 (Demonstration paper [Best Demonstration Runner Up Award]). [details] [pdf] |
[PVLDB] | Matteo Brucato, Miro Mannino, Azza Abouzied, Peter J. Haas, and Alexandra Meliou, sPaQLTooLs: A Stochastic Package Query Interface for Scalable Constrained Optimization, PVLDB, vol. 13, no. 12, 2020, pp. 2881ā2884 (Demonstration paper [Best Demonstration Award]). [details] [pdf] |
[PVLDB] | Xiaolan Wang and Alexandra Meliou, Explain3D: Explaining Disagreements in Disjoint Datasets, PVLDB, vol. 12, no. 7, 2019, pp. 779–792. [details] [pdf] |
[PVLDB] | Anna Fariha and Alexandra Meliou, Example-Driven Query Intent Discovery: Abductive Reasoning using Semantic Similarity, PVLDB, vol. 12, no. 11, 2019, pp. 1262–1275. [details] [pdf] |
[CACM] | Matteo Brucato, Azza Abouzied, and Alexandra Meliou, Scalable Computation of High-Order Optimization Queries, Communications of the ACM, vol. 62, no. 2, feb 2019, pp. 108–116 ([Research Highlight]). [details] [pdf] [url] [doi] |
[PVLDB] | Yue Wang, Alexandra Meliou, and Gerome Miklau, RC-Index: Diversifying Answers to Range Queries, PVLDB, vol. 11, no. 7, sep 2018, pp. 773–786. [details] [pdf] [doi] |
[DEBul] | Xiaolan Wang, Laura Haas, and Alexandra Meliou, Explaining Data Integration, IEEE Data Engineering Bulletin, vol. 41, no. 2, jun 2018, pp. 47–58. [details] [pdf] |
[VLDBJ] | Matteo Brucato, Azza Abouzied, and Alexandra Meliou, Package queries: efficient and scalable computation of high-order constraints, The VLDB Journal, vol. 27, no. 1, 2018 (Special Issue on Best Papers of VLDB 2016). [details] [pdf] [doi] |
[SIGMOD Record] | Matteo Brucato, Azza Abouzied, and Alexandra Meliou, A Scalable Execution Engine for Package Queries, SIGMOD Record, vol. 46, no. 1, mar 2017, pp. 24–31 ([ACM SIGMOD Research Highlight Award]). [details] [pdf] [doi] |
[PVLDB] | Yue Wang, Alexandra Meliou, and Gerome Miklau, Lifting the Haze off the Cloud: A Consumer-Centric Market for Database Computation in the Cloud, PVLDB, vol. 10, no. 4, 2016, pp. 373–384. [details] [pdf] [doi] |
[PVLDB] | Matteo Brucato, Juan Felipe Beltran, Azza Abouzied, and Alexandra Meliou, Scalable Package Queries in Relational Database Systems, PVLDB, vol. 9, no. 7, 2016, pp. 575–587 ([Best papers of VLDB 2016]). [details] [pdf] [doi] |
[PVLDB] | Xiaolan Wang, Mary Feng, Yue Wang, Luna Dong, and Alexandra Meliou, Error Diagnosis and Data Profiling with Data X-Ray, PVLDB, vol. 8, no. 12, 2015, pp. 1984–1987 (Demonstration paper). [details] [pdf] [doi] |
[PVLDB] | Cibele Freire, Wolfgang Gatterbauer, Neil Immerman, and Alexandra Meliou, A Characterization of the Complexity of Resilience and Responsibility for Self-join-free Conjunctive Queries, PVLDB, vol. 9, no. 3, 2015, pp. 180–191. [details] [pdf] [doi] |
[PVLDB] | Alexandra Meliou, Sudeepa Roy, and Dan Suciu, Causality and Explanations in Databases, PVLDB, vol. 7, no. 13, 2014, pp. 1715–1716 (Tutorial). [details] [pdf] [doi] |
[PVLDB] | Matteo Brucato, Rahul Ramakrishna, Azza Abouzied, and Alexandra Meliou, PackageBuilder: From Tuples to Packages, PVLDB, vol. 7, no. 13, 2014, pp. 1593–1596 (Demonstration paper). [details] [pdf] [doi] |
[PVLDB] | Alexandra Meliou, Wolfgang Gatterbauer, and Dan Suciu, Reverse Data Management, PVLDB, vol. 4, no. 11, 2011, pp. 1490–1493. [details] [pdf] |
[PVLDB] | Alexandra Meliou, Wolfgang Gatterbauer, Katherine F. Moore, and Dan Suciu, The Complexity of Causality and Responsibility for Query Answers and non-Answers, PVLDB, vol. 4, no. 1, 2010, pp. 34–45. [details] [pdf] |
[DEBul] | Alexandra Meliou, Wolfgang Gatterbauer, Joseph Y. Halpern, Christoph Koch, Katherine F. Moore, and Dan Suciu, Causality in Databases, IEEE Data Engineering Bulletin, vol. 33, no. 3, 2010, pp. 59–67. [details] [pdf] |
[InfSci] | Theodore Dalamagas, Alexandra Meliou, and Timos K. Sellis, Modeling and Manipulating the Structure of Hierarchical Schemas for the Web, Information Sciences, Elsevier, vol. 178, no. 4, 2008, pp. 985–1010. [details] [pdf] [doi] |
[ICDE] | Ke Yang and Alexandra Meliou, Non-Invasive Fairness in Learning through the Lens of Data Drift, in 40th IEEE International Conference on Data Engineering, 2024. [details] [pdf] |
[SIGMOD] | Maliha Tashfia Islam, Anna Fariha, Alexandra Meliou, and Babak Salimi, Through the Data Management Lens: Experimental Analysis and Evaluation of Fair Classification, in SIGMOD '22: International Conference on Management of Data, ACM, pp. 232–246. [details] [pdf] [url] [doi] |
[SIGMOD] | Sainyam Galhotra, Anna Fariha, Raoni Lourenço, Juliana Freire, Alexandra Meliou, and Divesh Srivastava, DataPrism: Exposing Disconnect between Data and Systems, in SIGMOD '22: International Conference on Management of Data, ACM, pp. 217–231. [details] [pdf] [url] [doi] |
[ICDT] | Raghavendra Addanki, Andrew McGregor, Alexandra Meliou, and Zafeiria Moumoulidou, Improved Approximation and Scalability for Fair Max-Min Diversification, in 25th International Conference on Database Theory (ICDT), Schloss Dagstuhl - Leibniz-Zentrum für Informatik, 2022, pp. 7:1–7:21. [details] [pdf] [url] [doi] |
[NewSum] | Nishant Yadav, Matteo Brucato, Anna Fariha, Oscar Yongquist, Julian Killingback, Alexandra Meliou, and Peter J. Haas, SubSumE: A Dataset for Subjective Summary Extraction from Wikipedia Documents, in New Frontiers in Summarization workshop (at EMNLP 2021). [details] [pdf] |
[ICDT] | Zafeiria Moumoulidou, Andrew McGregor, and Alexandra Meliou, Diverse Data Selection under Fairness Constraints, in International Conference on Database Theory, (ICDT), 2021, pp. 11:1–11:25. [details] [pdf] |
[SIGMOD] | Anna Fariha, Ashish Tiwari, Alexandra Meliou, Arjun Radhakrishna, and Sumit Gulwani, CoCo: Interactive Exploration of Conformance Constraints for Data Understanding and Data Cleaning, in Proceedings of the ACM SIGMOD International Conference on Management of Data (SIGMOD), 2021 (Demonstration paper). [details] [pdf] [doi] |
[SIGMOD] | Anna Fariha, Ashish Tiwari, Alexandra Meliou, Arjun Radhakrishna, and Sumit Gulwani, Conformance Constraint Discovery: Measuring Trust in Data-Driven Systems, in Proceedings of the ACM SIGMOD International Conference on Management of Data (SIGMOD), 2021 ([ACM SIGMOD Comprehensive Reproducibility Award]). [details] [pdf] [doi] |
[ICSE] | Brittany Johnson, Yuriy Brun, and Alexandra Meliou, Causal Testing: Understanding Defects' Root Causes, in Proceedings of the 42nd International Conference on Software Engineering (ICSE), 2020 ([ACM SIGSOFT Distinguished Artifact Award]). [details] [pdf] [doi] |
[PODS] | Cibele Freire, Wolfgang Gatterbauer, Neil Immerman, and Alexandra Meliou, New Results for the Complexity of Resilience for Binary Conjunctive Queries with Self-Joins, in Symposium on Principles of Database Systems (PODS), 2020, pp. 271–284. [details] [pdf] [doi] |
[SIGMOD] | Anna Fariha, Suman Nath, and Alexandra Meliou, Causality-Guided Adaptive Interventional Debugging, in Proceedings of the ACM SIGMOD International Conference on Management of Data (SIGMOD), 2020, pp. 431–446. [details] [pdf] [doi] |
[SIGMOD] | Matteo Brucato, Nishant Yadav, Azza Abouzied, Peter Haas, and Alexandra Meliou, Stochastic Package Queries in Probabilistic Databases, in Proceedings of the ACM SIGMOD International Conference on Management of Data (SIGMOD), 2020, pp. 269–283. [details] [pdf] [doi] |
[ICDE] | Xiaolan Wang, Luna Dong, Yang Li, and Alexandra Meliou, MIDAS: Finding the Right Web Sources to Fill Knowledge Gaps, in 35th IEEE International Conference on Data Engineering, 2019, pp. 578–589. [details] [pdf] |
[SIGMOD] | Anna Fariha, Sheikh Muhammad Sarwar, and Alexandra Meliou, SQuID: Semantic Similarity-Aware Query Intent Discovery, in Proceedings of the ACM SIGMOD International Conference on Management of Data (SIGMOD), 2018, pp. 1745–1748 (Demonstration paper). [details] [pdf] [doi] |
[ESEC/FSE] | Yuriy Brun and Alexandra Meliou, Software Fairness, in Proceedings of the New Ideas and Emerging Results Track at the 26th ACM Joint European Software Engineering Conference and Symposium on the Foundations of Software Engineering (ESEC/FSE), 2018, pp. 754–759. [details] [pdf] [doi] |
[ESEC/FSE] | Rico Angell, Brittany Johnson, Yuriy Brun, and Alexandra Meliou, Themis: Automatically Testing Software for Discrimination, in Proceedings of the Demonstrations Track at the The 26th ACM Joint European Software Engineering Conference and Symposium on the Foundations of Software Engineering (ESEC/FSE), 2018, pp. 871–875 (Demonstration paper). [details] [pdf] [doi] |
[EDBT] | Haopeng Zhang, Yanlei Diao, and Alexandra Meliou, EXStream: Explaining Anomalies in Event Stream Monitoring, in 20th International Conference on Extending Database Technology (EDBT), 2017, pp. 156–167. [details] [pdf] [doi] |
[SIGMOD] | Xiaolan Wang, Alexandra Meliou, and Eugene Wu, QFix: Diagnosing errors through query histories, in Proceedings of the ACM SIGMOD International Conference on Management of Data (SIGMOD), 2017, pp. 1369–1384. [details] [pdf] [doi] |
[ESEC/FSE] | Sainyam Galhotra, Yuriy Brun, and Alexandra Meliou, Fairness Testing: Testing Software for Discrimination, in Proceedings of 2017 11th Joint Meeting of the European Software Engineering Conference and the ACM SIGSOFT Symposium on the Foundations of Software Engineering, pp. 498–510 ([ACM SIGSOFT Distinguished Paper Award]). [details] [pdf] [doi] |
[SIGMOD] | Xiaolan Wang, Alexandra Meliou, and Eugene Wu, QFix: Demonstrating error diagnosis in query histories, in Proceedings of the ACM SIGMOD International Conference on Management of Data (SIGMOD), 2016, pp. 2177–2180 (Demonstration paper). [details] [pdf] [doi] |
[SIGMOD] | Xiaolan Wang, Xin Luna Dong, and Alexandra Meliou, Data X-Ray: A Diagnostic Tool for Data Errors, in Proceedings of the ACM SIGMOD International Conference on Management of Data (SIGMOD), 2015, pp. 1231–1245. [details] [pdf] [doi] |
[ISSTA] | Kıvanç Muşlu, Yuriy Brun, and Alexandra Meliou, Preventing Data Errors with Continuous Testing, in Proceedings of the ACM SIGSOFT International Symposium on Software Testing and Analysis (ISSTA), 2015, pp. 373–384. [details] [pdf] [doi] |
[SIGMOD] | Kevin Fernandes, Matteo Brucato, Rahul Ramakrishna, Azza Abouzied, and Alexandra Meliou, PackageBuilder: querying for packages of tuples, in Proceedings of the ACM SIGMOD International Conference on Management of Data (SIGMOD), 2014, pp. 1613–1614 (Undergraduate research poster). [details] [pdf] [doi] |
[SIGMOD] | Ravali Pochampally, Anish Das Sarma, Xin Luna Dong, Alexandra Meliou, and Divesh Srivastava, Fusing Data with Correlations, in Proceedings of the ACM SIGMOD International Conference on Management of Data (SIGMOD), 2014, pp. 433–444. [details] [pdf] [doi] |
[DATA4U] | Matteo Brucato, Azza Abouzied, and Alexandra Meliou, Improving Package Recommendations Through Query Relaxation, in Proceedings of the 1st International DATA4U Workshop, in conjunction with VLDB, 2014. [details] [pdf] [doi] |
[ESEC/FSE] | Kıvanç Muşlu, Yuriy Brun, and Alexandra Meliou, Data Debugging with Continuous Testing, in Proceedings of the New Ideas Track at the 9th Joint Meeting of the European Software Engineering Conference and ACM SIGSOFT Symposium on the Foundations of Software Engineering (ESEC/FSE13), 2013, pp. 631–634. [details] [pdf] [doi] |
[SIGMOD] | Alexandra Meliou, Yisong Song, and Dan Suciu, Tiresias: A Demonstration of How-To Queries, in Proceedings of the ACM SIGMOD International Conference on Management of Data (SIGMOD), 2012, pp. 709–712 (Demonstration paper [Best Demonstration Award]). [details] [pdf] [doi] |
[SIGMOD] | Alexandra Meliou and Dan Suciu, Tiresias: The Database Oracle for How-To Queries, in Proceedings of the ACM SIGMOD International Conference on Management of Data (SIGMOD), 2012, pp. 337–348. [details] [pdf] [doi] |
[TaPP] | Wolfgang Gatterbauer, Alexandra Meliou, and Dan Suciu, Default-all is Dangerous!, in 3rd USENIX Workshop on the Theory and Practice of Provenance (TaPP), 2011. [details] [pdf] |
[TaPP] | Alexandra Meliou, Wolfgang Gatterbauer, and Dan Suciu, Bringing Provenance to its Full Potential Using Causal Reasoning, in 3rd USENIX Workshop on the Theory and Practice of Provenance (TaPP), 2011. [details] [pdf] |
[SIGMOD] | Alexandra Meliou, Wolfgang Gatterbauer, Suman Nath, and Dan Suciu, Tracing Data Errors with View-Conditioned Causality, in Proceedings of the ACM SIGMOD International Conference on Management of Data (SIGMOD), 2011, pp. 505–516. [details] [pdf] [doi] |
[NIPS] | Abhay Jha, Vibhav Gogate, Alexandra Meliou, and Dan Suciu, Lifted Inference Seen from the Other Side: The Tractable Features, in 24th Annual Conference on Neural Information Processing Systems (NIPS), 2010, pp. 973–981. [details] [pdf] |
[MUD] | Alexandra Meliou, Wolfgang Gatterbauer, Katherine F. Moore, and Dan Suciu, Why So? or Why No? Functional Causality for Explaining Query Answers, in Proceedings of the 4th International VLDB workshop on Management of Uncertain Data (MUD) in conjunction with VLDB, 2010, pp. 3–17. [details] [pdf] |
[AMW] | Alexandra Meliou, Carlos Guestrin, and Joseph M. Hellerstein, Multiresolution Cube Estimators for Sensor Network Aggregate Queries, in Proceedings of the 4th Alberto Mendelzon International Workshop on Foundations of Data Management (AMW), 2010. [details] [pdf] |
[IPSN] | Alexandra Meliou, Carlos Guestrin, and Joseph M. Hellerstein, Approximating Sensor Network Queries using In-Network Summaries, in Proceedings of the 8th International Conference on Information Processing in Sensor Networks (IPSN), 2009, pp. 229–240. [details] [pdf] |
[AAAI] | Alexandra Meliou, Andreas Krause, Carlos Guestrin, and Joseph M. Hellerstein, Nonmyopic Informative Path Planning in Spatio-Temporal Models, in Proceedings of the 22nd National Conference on Artificial Intelligence (AAAI), 2007, pp. 602–607. [details] [pdf] |
[IPSN] | Alexandra Meliou, David Chu, Joseph M. Hellerstein, Carlos Guestrin, and Wei Hong, Data Gathering Tours in Sensor Networks, in Proceedings of the 5th International Conference on Information Processing in Sensor Networks (IPSN), 2006, pp. 43–50. [details] [pdf] [doi] |
[PhD] | Alexandra Meliou, Querying Uncertain Data in Resource Constrained Settings, Ph.D. dissertation, University of California, Berkeley, Berkeley, CA, 2009. [details] [pdf] |
[MSc] | Alexandra Meliou, Data Gathering Tours in Sensor Networks, Master's thesis, University of California, Berkeley, Berkeley, CA, 2005. [details] [pdf] |
[BSc] | Alexandra Meliou, Modeling and Exploring the Algebraic Properties of Hierarchical Structures, Athens, Greece, 2003. [details] [pdf] |
Teaching
- Spring 2023 COMPSCI 345: Practice and Applications of Data Management
- Fall 2022 COMPSCI 345: Practice and Applications of Data Management
- Spring 2020 COMPSCI 345: Practice and Applications of Data Management
- Fall 2019 COMPSCI 345: Practice and Applications of Data Management
- Fall 2019 COMPSCI H345: Practice and Applications of Data Management - Honors colloquium
- Spring 2019 COMPSCI 345: Practice and Applications of Data Management
- Fall 2018 COMPSCI 345: Practice and Applications of Data Management
- Spring 2018 CMPSCI 645: Database Design and Implementation
- Fall 2017 COMPSCI 345: Practice and Applications of Data Management
- Fall 2017 COMPSCI H345: Practice and Applications of Data Management - Honors colloquium
- Spring 2017 CMPSCI 645: Database Design and Implementation
- Fall 2015 CMPSCI 345: Practice and Applications of Data Management
- Spring 2015 CMPSCI 645: Database Design and Implementation
- Fall 2014 CMPSCI 345: Practice and Applications of Data Management
- Fall 2014 CMPSCI H345: Practice and Applications of Data Management - Honors section
- Fall 2013 CMPSCI 390DB: Practice and Applications of Data Management
- Fall 2013 CMPSCI H390DB: Practice and Applications of Data Management - Honors section
- Spring 2013 CMPSCI 390DB: Practice and Applications of Data Management
- Fall 2012 CMPSCI 645: Database Design and Implementation
- Winter 2011 CSE444: Introduction to Database Systems (University of Washington)
- Winter 2010 CSE590q: Positive and Negative Provenance in Database Systems (seminar, University of Washington)
Current PhD students and postdocs:
- Riddho Ridwanul Haque (PhD, currently on leave at Google)
- Iro Moumoulidou (PhD)
- Vasilis Vittis (PhD)
Graduated PhD students and postdocs:
- Matteo Brucato (PhD, 2021; co-advised with Peter Haas) Senior Researcher, Microsoft Research
- Anna Fariha (PhD, 2021) Assistant Professor, University of Utah (previously, Researcher, Microsoft PROSE team)
- Xiaolan Wang (PhD, 2018) Software Engineer, Meta (previously, Senior Research Scientist, Megagon Labs)
- Yue Wang (PhD, 2017; co-advised with Gerome Miklau) Senior Research SDE, Microsoft Research.
- Ke Yang (Postdoc) Assistant Professor, University of Texas San Antonio
Other alumni:
- Maliha Islam (MS, Software Engineer, Microsoft)
- Ravali Pochampally (MS, now at Google)
- Rahul Ramakrishna (MS, Fall 2013-Spring 2014)
- Kevin Fernandes (undergraduate, Spring 2014, now M.D Candidate at Albert Einstein College of Medicine)
- Jeffrey Pezzone (undergraduate, Spring 2013, now at CISCO Systems)
- Joseph Scherr (undergraduate, REU summer 2013)
- Hridya Turlapati (undergraduate, Spring 2013)
- Linda Yeboah (undergraduate, Summer 2014)
Bio
Alexandra Meliou is an Associate Professor in the College of Information and Computer Sciences, at the University of Massachusetts Amherst. Prior to joining UMass, she was a Postdoctoral Research Associate at the University of Washington. Alexandra received her PhD degree from the Electrical Engineering and Computer Sciences Department at the University of California, Berkeley. She has received recognitions for research, teaching, and service, including a CACM Research Highlight, an ACM SIGMOD Research Highlight Award, an ACM SIGSOFT Distinguished Paper Award, an NSF CAREER Award, a Google Faculty Research Award, multiple Distinguished Reviewer Awards, and a Lilly Fellowship for Teaching Excellence. Her research focuses on data provenance, causality, explanations, data quality, and algorithmic fairness.
University of California, Berkeley
PhD in Computer Science (December 2009)
MS in Computer Science (December 2005)
Advisors: Joseph Hellerstein and Carlos Guestrin
National Technical University of Athens
BS in Electrical Engineering and Computer Science (June 2003)
Advisor: Timos Sellis
Service
Conference Organization and Editorial Service
- PC co-Chair for SIGMOD 2024
- Diversity and Inclusion Chair for ICDE 2023
- Workshop Chair for VLDB 2021
- Tutorials Chair for ICDE 2021
- Associate editor for VLDBJ (2019-2025).
- Associate editor for PVLDB 2020.
- Associate editor for PVLDB 2019.
- Associate editor for the IEEE Data Engineering Bulletin (2018-2019).
- PC co-Chair for FairWare 2018.
- PC co-Chair for ICDE PhD Symposium 2018.
- PC co-Chair for WebDB 2017.
- PC Track Chair for SIGMOD 2016.
- New Researcher Symposium co-Chair for SIGMOD 2015.
- Student Travel Award selection committee co-Chair for SIGMOD 2015.
- New Researcher Symposium co-Chair for SIGMOD 2014.
- Student Travel Award selection committee co-Chair for SIGMOD 2014.
- Program Chair for TaPP 2013
- Demonstration Chair for SSDBM 2013
- Undergraduate Research Program co-Chair for SIGMOD 2013.
Program Committees
- Proceedings of the VLDB Endowment (PVLDB) 2023.
- Conference on Management of Data (SIGMOD) 2023.
- Conference on Management of Data (SIGMOD) 2021 (Associate Editor).
- Conference on Management of Data (SIGMOD) 2020.
- Hellenic Database Management Symposium 2019.
- Alberto Mendelzon International Workshop on Foundations of Data Management (AMW) 2019.
- Conference on Management of Data (SIGMOD) 2019. (Core PC)
- Proceedings of the VLDB Endowment (PVLDB) 2018.
- Conference on Management of Data (SIGMOD) 2018.
- Conference on Management of Data (SIGMOD) 2017.
- Alberto Mendelzon International Workshop on Foundations of Data Management (AMW) 2017.
- International Conference on Data Engineering (ICDE) 2016.
- Conference on Management of Data (SIGMOD) 2015.
- Proceedings of the VLDB Endowment (PVLDB) 2015.
- VLDB PhD Workshop 2015.
- International Conference on Scientific and Statistical Database Management (SSDBM) 2014, demo track.
- Proceedings of the VLDB Endowment (PVLDB) 2014.
- Conference on Management of Data (SIGMOD) 2014, demo track.
- Conference on Very Large Databases (VLDB) 2013, demo track.
- Conference on Management of Data (SIGMOD) 2013.
- International Conference on Data Engineering (ICDE) 2013.
- International Conference on Information and Knowledge Management (CIKM) 2012.
- Conference on Management of Data (SIGMOD) 2012, demo track.
- International Conference on Data Engineering (ICDE) 2012.
- Workshop on the Theory and Practice of Provenance (TaPP) 2012.
- Workshop on the Web and Databases (WebDB) 2012.
- Proceedings of the VLDB Endowment (PVLDB) 2011.
- Conference on Management of Data (SIGMOD) 2011.
- Workshop on Management of Uncertain Data (MUD) 2011.
Other Service
- Core member of D&I in DB
- ACM Publications Board Task Force on Improving Peer-Reviewer Incentives.
- ACM SIGMOD Jim Gray Dissertation Award (2019 and 2020).
Personal
My sister is a doctor of internal medicine, specializing in infectious diseases.