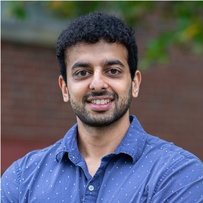
mohit iyyer
miyyer@cs.umass.edu // CV // Scholar // github // twitter
I am an associate professor in computer science at UMass Amherst and a member of UMass NLP. Previously, I was a Young Investigator at AI2; before that, I completed my PhD at the University of Maryland, College Park, advised by Jordan Boyd-Graber and Hal Daumé III.
My research interests lie broadly in natural language processing and machine learning. Problems that I'm currently excited about include:
(1) Improving instruction following abilities of large language models for long-form generation
(2) Designing methods to evaluate long-form & multilingual text (e.g., for factuality and coherence)
(3) Building collaborative human-LLM systems to help human authors in creative writing tasks
(4) Increasing robustness of LLM-generated text detectors to attacks (e.g., paraphrasing)